The ACM TURC 2017 (SIGMOD China) conference is a new leading international forum for database researchers, practitioners, developers, and users to explore cutting-edge ideas and results, and to exchange techniques, tools, and experiences. We invite the submission of original research contributions relating to all aspects of data management defined broadly, and particularly encourage submissions on topics of emerging interest in the research and development communities.
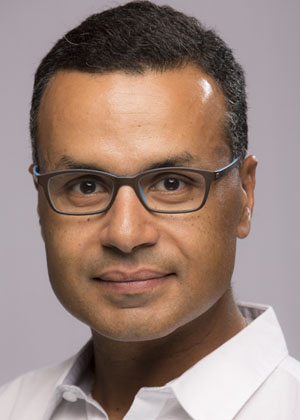
|
|
Ihab Francis Ilyas (University of Waterloo, Canada)
Title: Building Scalable Machine Learning Solutions for Data Curation
Abstract: Machine learning tools promise to help solve data curation problems. While the principles are well understood, the engineering details in configuring and deploying ML techniques are the biggest hurdle. In this talk I discuss why leveraging data semantics and domain-specific knowledge is key in delivering the optimizations necessary for truly scalable ML curation solutions. The talk focuses on two main problems: (1) entity consolidation, which is arguably the most difficult data curation challenge because it is notoriously complex and hard to scale; and (2) using probabilistic inference to suggest data repair for identified errors and anomalies using our new system called HoloCLean. Both problems have been challenging researchers and practitioners for decades due to the fundamentally combinatorial explosion in the space of solutions and the lack of ground truth. There’s a large body of work on this problem by both academia and industry. Techniques have included human curation, rules-based systems, and automatic discovery of clusters using predefined thresholds on record similarity Unfortunately, none of these techniques alone has been able to provide sufficient accuracy and scalability. The talk aims at providing deeper insight into the entity consolidation and data repair problems and discusses how machine learning, human expertise, and problem semantics collectively can deliver a scalable, high-accuracy solution.
Bio: Ihab Ilyas is a professor in the Cheriton School of Computer Science at the University of Waterloo, where his main research focuses on the areas of big data and database systems, with special interest in data quality and integration, managing uncertain data, rank-aware query processing, and information extraction. Ihab is also a co-founder of Tamr, a startup focusing on large-scale data integration and cleaning. He is a recipient of the Ontario Early Researcher Award (2009), a Cheriton Faculty Fellowship (2013), an NSERC Discovery Accelerator Award (2014), and a Google Faculty Award (2014), and he is an ACM Distinguished Scientist. Ihab is an elected member of the VLDB Endowment board of trustees, elected SIGMOD vice chair, and an associate editor of the ACM Transactions of Database Systems (TODS). He holds a PhD in computer science from Purdue University, West Lafayette. |
|
|
|
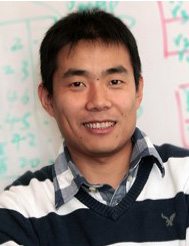
|
|
Feifei Li (University of Utah, USA)
Title: Towards a Shared-Everything Database on Distributed Log-Structured Storage
Abstract: Efficient transaction processing over a large database is a key requirement for many mission-critical applications. Though modern data management systems have achieved good performance through horizontal partitioning, their performance deteriorates for cross-partition distributed transaction processing. This paper presents Solar, a distributed shared- everything relational database system on a cluster with nodes interconnected on a common Ethernet network. Solar achieves high performance transaction processing without relying on any particular properties of the underlying workloads. The system consists of an in-memory transaction engine, a distributed storage engine and an elastic processing engine. Its key features include: 1) a shared-everything architecture based on a two-layer log-structured merge-tree design; 2) a new concurrency control algorithm designed to work with the log-structured storage, which ensures efficient and non-blocking transaction processing even when the storage layer is compacting data among nodes in the background; 3) fine-grained data access algorithms to effectively adjust and balance network communications within the cluster. The system is empirically compared against existing distributed database systems, such as VoltDB, MySQL-Cluster, and Tell, using several benchmarks, including TPC-C, Smallbank and E- commerce benchmarks. Experimental results have demonstrated that Solar has clearly outperformed other systems. Solar has been successfully deployed at the Bank of Communications, one of the largest commercial banks in China.
Bio: Feifei Li is currently a professor at the School of Computing, University of Utah. He obtained his Bachelor's degree from Nanyang Technological University (transferred from Tsinghua University) in 2001 and PhD from Boston University in 2007. His research focuses on improving the scalability, efficiency, and effectiveness of data analytics and large-scale data management systems. He also works on data security problems in these systems. He was a recipient for a NSF career award in 2011, two HP IRP awards in 2011 and 2012 respectively, a Google App Engine award in 2013, IEEE ICDE best paper award in 2004, IEEE ICDE 10+ Years Most Influential Paper Award in 2014, a Google Faculty award in 2015, SIGMOD Best Demonstration Award in SIGMOD 2015, SIGMOD 2016 Best Paper Award SIGMOD Research Highlight Award in 2017, a VISA research faculty award in 2017, and an oversea key research collaboration project on big data award by NSFC in 2017. He is/was the demo PC co-chair for SIMGOD 2018, a senior PC member for SIGMOD 2019, a member of the SIGMOD Jim Gray Dissertation Award selection committee in 2017 and 2018, a member of the CIKM 2017 best paper award selection committee, a PC area chair for SIGMOD 2015 and ICDE 2014, the demo PC co-chair for VLDB 2014, and the general co-chair for SIGMOD 2014. He currently serves as an associate editor for ACM TODS, IEEE TKDE, and DAPD by Springer. |
|
|
|
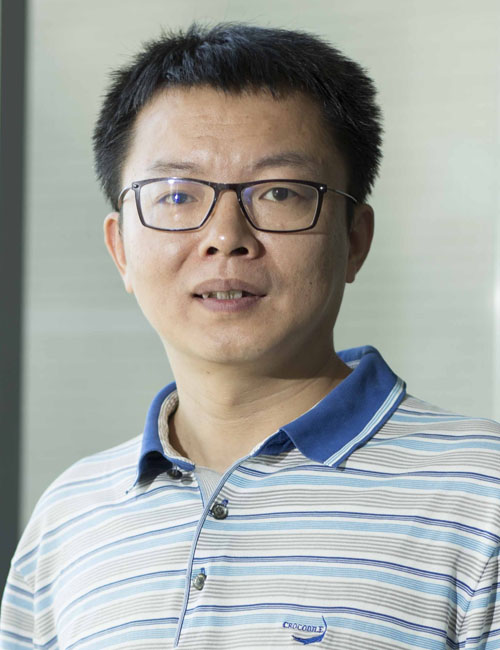
|
|
Bin Cui (Beijing University)
Title: System Design for Distributed Machine Learning
Abstract: Distributed machine learning has been extensively studied to meet the explosive increase of data volume and model size. There are several critical concerns in designing an efficient distributed machine learning system, e.g., parallelism, synchronization, and data communication. In this talk, I will introduce our work trying to optimize distributed machine learning system in these aspects, e.g., the hybrid parallel method to combine the merits of data parallel and model parallel paradigms, the heterogeneity-aware synchronization protocol, and the data sketch algorithm to compress the gradients. I will also introduce our distributed ML system, named Angel, which can facilitate the development of large-scale ML applications in production environment. Angel has been deployed in a Tencent production cluster with thousands of nodes and supports various applications (https://github.com/Tencent/angel).
Bio: Bin Cui is a professor in the School of EECS and Director of Institute of Network Computing and Information Systems, at Peking University. His research interests include database system architectures, big data management and analytics. He has published over 100 research articles in international journals and conference proceedings. He has served in the Technical Program Committee of various international conferences including SIGMOD, VLDB, ICDE and KDD, and as Vice PC Chair of ICDE 2011 & 2018, Demo Co-Chair of ICDE 2014, Area Chair of VLDB 2014, PC Co-Chair of APWeb 2015 and WAIM 2016. He is serving as a Trustee Board Member of VLDB Endowment, is/was also in the Editorial Board of TKDE, VLDB Journal, Distributed and Parallel Databases Journal, and Information Systems. He was awarded Microsoft Young Professorship award (MSRA 2008), CCF Young Scientist award (2009), Second Prize of Natural Science Award of MOE China (2014), and appointed as Cheung Kong distinguished Professor by MOE in 2016. |
|
|
|
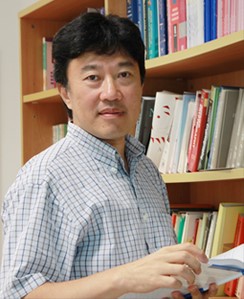
|
|
Jeffrey Xu Yu (The Chinese University of Hong Kong)
Title: Graph Processing: The Integration of RDBMS and Graph System
Abstract: To support analytics on massive graphs such as online social networks, RDF, Semantic Web, etc. many new graph algorithms are designed to query graphs for a specific problem, and many distributed graph processing systems are developed to support graph querying by programming. In this talk, first, we focus on RDBM, which has been well studied over decades to manage large datasets. We revisit the issue how RDBMS can support graph processing at the SQL level. Our work is motivated by the fact that there are many relations stored in RDBMS that are closely related to a graph in real applications and need to be used together to query the graph, and RDBMS is a system that can query and manage data while data may be updated over time. To support graph processing, we propose 4 new relational algebra operations. The 4 new relational algebra operations can be defined by the 6 basic relational algebra operations with group-by-&-aggregation. We revisit SQL recursive queries and show that the 4 operations with others are ensured to have a fixpoint, following the techniques studied in Datalog, and enhance the recursive WITH clause in SQL'99. Such enhanced recursive WITH Clause can be supported by major RDBMSs. Second, we discuss how to translate such newly introduced operations into a graph system to achieve efficiency.
Bio: Dr Jeffrey Xu Yu is a Professor in the Department of Systems Engineering and Engineering Management, The Chinese University of Hong Kong. His current main research interests include graph mining, graph query processing, graph pattern matching, keywords search in databases, and online social networks. Dr. Yu served as an Information Director and a member in ACM SIGMOD executive committee (2007-2011), an associate editor of IEEE Transactions on Knowledge and Data Engineering (2004-2008), and an associate editor in VLDB Journal (2007-2013). Currently he servers as an associate editor of ACM Transactions on Database Systems (TODS), WWW Journal, Data Science and Engineering, the International Journal of Cooperative Information Systems, the Journal on Health Information Science and Systems (HISS), and Journal of Information Processing. Dr. Yu served/serves in many organization committees and program committees in international conferences/workshops including PC Co-chair of APWeb'04, WAIM'06, APWeb/WAIM'07, WISE'09, PAKDD'10, DASFAA'11, ICDM'12, NDBC'13, ADMA'14, CIKM'15 and Bigcomp17, and conference general Co-chair of APWeb'13 and ICDM'18. |
|
|
|
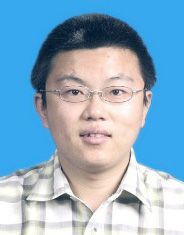
|
|
Guoliang Li (Tsinghua University)
Title: Human-in-the-loop Data Integration
Abstract: Data integration aims to integrate data in different sources and provide users with a unified view. However, data integration cannot be completely addressed by purely automated methods. In this talk, I present a hybrid human-machine data integration framework that harnesses human ability to address this problem, and especially focus on the problem of entity matching. The framework first uses rule-based algorithms to identify possible matching pairs and then utilizes the crowd to refine these candidate pairs in order to compute actual matching pairs. In the first step, I introduce similarity-based rules and knowledge-based rules to obtain some candidate matching pairs, and develop effective algorithms to learn these rules based on some given positive and negative examples. I also introduce our distributed in-memory system DIMA to efficiently apply these rules. In the second step, I present a selection-inference-refine framework that uses the crowd to verify the candidate pairs, which first selects some “beneficial” tasks to ask the crowd and then uses transitivity and partial order to infer the answers of unasked tasks based on the crowdsourcing results of the asked tasks. I introduce our crowd-powered database system CDB that allows users to utilize a SQL-like language for processing crowd-based queries. Lastly, I provide emerging challenges in human-in-the-loop data integration.
Bio: Guoliang Li is an Associate Professor of Department of Computer Science, Tsinghua University, Beijing, China. His research interests include crowdsourced data management, big spatio-temporal data analytics, large-scale data cleaning and integration. He has published more than 100 papers in premier conferences and journals, such as SIGMOD, VLDB, ICDE, SIGKDD, SIGIR, TODS, VLDB Journal, and TKDE. He is a PC co-chair of WAIM 2014, WebDB 2014, and NDBC 2016. He servers as associate editor for IEEE Transactions and Data Engineering, VLDB Journal, ACM Transactions on Data Science, IEEE Data Engineering Bulletin, ACM Journal of Data and Information Quality, and BigData Research. He has regularly served as the PC members of many premier conferences, such as SIGMOD, VLDB, KDD, ICDE, WWW, IJCAI, and AAAI. His papers have been cited more than 4500 times. He received VLDB Early Research Contribution Award 2017, IEEE TCDE Early Career Award 2014, The National Youth Talent Support Program 2017, ChangJiang Young Scholar 2016, NSFC Excellent Young Scholars Award 2014, CCF Young Scientist 2014. He received CIKM 2017 Best Paper Award, APWeb 2014 Best Paper Award, DASFAA 2014 Best Paper Runner-up. |
|
|
|
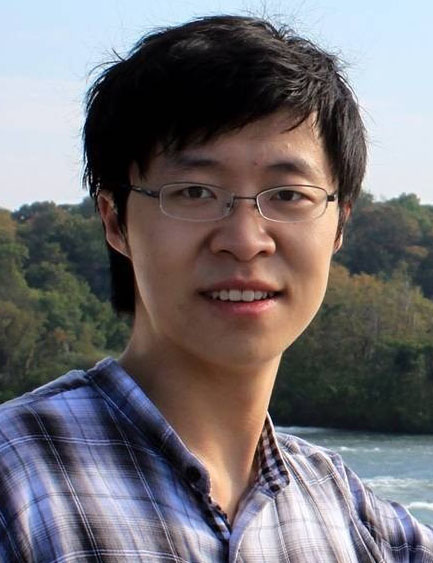
|
|
Ju Fan (Renmin University)
Title: Incentive-Based Entity Collection using Crowdsourcing
Abstract: Crowdsourced entity collection leverages human’s ability to collect entities that are missing in a database, which has many real-world applications, such as knowledge base enrichment and enterprise data collection. There are several challenges. First, it is hard to evaluate the workers’ quality because a worker’s quality depends on not only the correctness of her provided entities but also the distinctness of these entities compared with the collected ones by other workers. Second, crowd workers are likely to provide popular entities and different workers will provide many duplicated entities, leading to a waste of money and low coverage. In this talk, we propose an incentive-based crowdsourced entity collection framework CrowdEC that encourages workers to provide more distinct items using an incentive strategy. CrowdEC proposes a worker model and evaluates a worker’s quality based on cross validation and entity checking. CrowdEC devises a worker utility model that considers both worker’s quality and entities’ distinctness provided by workers. CrowdEC proposes a worker elimination method to block workers with a low utility, which solves the first challenge. On the other hand, CrowdEC proposes an incentive pricing technique that encourages each qualified (i.e., non-eliminated) worker to provide distinct entities rather than duplicates.
Bio: Ju FAN is an associate professor at Renmin University of China. He received his Ph.D. from Tsinghua University in 2012, and worked as a research fellow at School of Computing, National University of Singapore from 2012 to 2015. His research interests are in general area of database, with emphasis on the topics including crowdsourced data management and data integration. He has published about 20 papers on top conferences/journals (CCF-A), including SIGMOD, VLDB, ICDE and TKDE. He served as a PC member for VLDB 2018 and ACM Multimedia 2015, and a reviewer for VLDB Journal and IEEE TKDE. He is also a recipient of ACM China Rising Star award. |
|
|
|
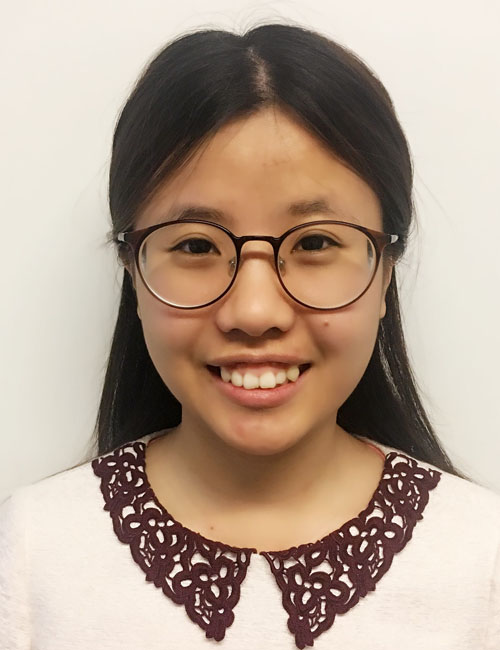
|
|
Lu Chen (Aalborg University, Denmark)
Title:Indexing and Querying Metric Spaces
Abstract: With the rapid developments of computer, Internet, communicational and positioning technologies, the volume of the data is increasing rapidly resulting from the scientific computing, the social life and the industrial production. The data is high dimensional, multi-source, heterogeneous, uncertain, and incomplete. Hence, we need a more generic model, i.e., the metric space. Metric spaces can support various data types and flexible distance metrics, and thus, they are more useful. The speaker systematically explores indexing and query processing technologies in metric spaces, including metric index structures, metric query processing, and metric query usability
Bio: Lu Chen is an Assistant Professor in Aalborg University, Denmark. She received the PHD degree in Computer Science from Zhejiang University, China in 2016, and then worked as a research fellow in Nanyang Technology University from Oct. 2016 to Sep. 2017. Her research concerns data management and data-intensive systems, and its focus is on metric data management. Lu has published more than 20 papers on top/important database conferences (e.g., SIGMOD, VLDB, ICDE, SIGIR, DASFAA) and journals (e.g., VLDBJ, TKDE, Information Sciences). Her paper was selected as one of best papers in ICDE 2015, and CCF selects her thesis as one of the excellent PHD theses. She was also a publication chair of WISE 2017. |
|
|
|