ACM TURC 2019 (SIGAI CHINA)
The ACM Turing Celebration Conference - China (ACM TURC 2019), will be held at May 17-20, 2019, Chengdu, Sichuan, with its key theme on "Interconnected, Inspired, intelligent (注智世界,赋能未来)". The conference serves as a highly selective and premier international forum on com-puter science research. In honor of Alan Turing, founder of computer logic and father of computer science, the Association for Computing Machinery (ACM) established A. M. Turing Award in 1966 to award individuals with remarkable contributions to the computer industry. The Turing Award is recognized as the highest distinction in computer science and the Nobel Prize of computing.
For the past few years, the ACM Symposium on Artificial Intelligence has been a primary gather-ing forum for promote and support the growth and application of AI principles and techniques throughout computing around the world. ACM SIGAI CHINA symposium, co-located with ACM TURC 2019, aims to provide a world’s premier forum of renowned researchers to share their in-sightful opinions, discuss the upcoming challenges and promote the development of Artificial In-telligence.
会议日程
2019-05-18 : 百合厅A (Lily Hall A) |
Time |
Talks |
14:00-14:40
|
特邀报告
1
Keynote 1 |
题目:面向深度NLP建模的张量乘积生成网络
Title :Tensor Product Generation Networks
for Deep NLP Modeling |
演讲者 吴大鹏
Speaker
Dapeng Wu |
主持人 左旺孟
Chair Wangmeng Zuo |
14:40- 15:20
|
特邀报告
2
Keynote 2 |
题目:跨媒体智能
Title :Cross-media intelligence |
演讲者 申恒涛
Speaker Heng Tao Shen |
主持人 左旺孟
Chair Wangmeng Zuo |
15:20 - 16:00
|
特邀报告
3
Keynote 3 |
题目:基于有限样本数据的深度神经网络学习
Title :Learning Deep Neural Networks from Limited Data |
演讲者 原田逹也
Speaker Tatsuya Harada |
主持人 左旺孟
Chair Wangmeng Zuo |
16:00 -
16:10
|
茶歇
Tea Break |
16:10 - 16:50
|
特邀报告 4
Keynote 4 |
题目:基于AI的临床骨龄评估计算机辅助系统
Title :A computer-aided system using artificial intelligence for clinical
bone age assessment |
演讲者 蔡辅仁
Speaker Fuu-Jen Tsai |
主持人 姬艳丽
Chair Yanli Ji |
16:50 - 17:30
|
特邀报告
5
Keynote 5 |
题目:医疗AI中的图像识别
Title :Image Recognition in Healthcare |
演讲者 俞益洲
Speaker Yizhou Yu |
主持人 姬艳丽
Chair
Yanli Ji |
2019-05-19 : 百合厅A (Lily Hall A) |
Time |
Talks |
14:00- 14:40
|
特邀报告
1
Keynote 1 |
题目:当表达学习遇上知识推理:AI新趋势
Title:Representation
Learning Meets Knowledge Reasoning: New Trends in AI |
演讲者 林倞
Speaker Liang Lin |
主持人 王旗龙
Chair Qilong Wang |
14:40- 15:20
|
特邀报告
2
Keynote 2 |
题目: 面向边缘AI的深度神经网络学习量化机制
Title Towards Edge AI:Learned Quantization for Highly Accurate Binary Neural
Network |
演讲者 华刚
Speaker Gang Hua |
主持人 林倞
Chair Liang Lin |
15:20- 16:00
|
特邀报告
3
Keynote 3 |
题目:开放域知识抽取研究新进展
Title :Open
Domain Knowledge Extraction |
演讲者 刘康
Speaker Kang Liu |
主持人
王旗龙
Chair Qilong Wang
|
16:00 -
16:10
|
茶歇
Tea Break |
16:10- 16:50
|
特邀报告 4
Keynote 4 |
题目:基于深度图推理的可解释性AI
Title:Explainable AI with Deep Graph reasoning |
演讲者 梁小丹
Speaker Xiaodan Liang |
主持人
王旗龙
Chair Qilong Wang
|
16:50 - 17:20
|
专题研讨会: 最佳论文论坛
Session: Best paper Forum |
主持人
梁小丹
Chair Xiaodan Liang
|
最佳论文分享:
DTR-GAN: Dilated Temporal Relational Adversarial Network for Video
Summarization(Speaker:Yujia
Zhang)
VRGym: A Virtual Testbed for Physical and
Interactive A(Speaker:
Xu Xie) |
17:20
- 17:40
|
最佳论文颁奖
Best paper reward |
|
|
Organization Committee
General Co-Chairs:
Liang Lin, Sun Yat-sen University
Wangmeng Zuo, Harbin Institute of Technology
Program Chairs:
Xiaodan Liang, Sun Yat-sen University
Yanli Ji, University of Electronic Science and Technology of China
Public Chairs:
Yan Gao, DMAI Inc.
Qilong Wang, Tianjin University
特邀报告
蔡辅仁
(中国中医药大学副校长)
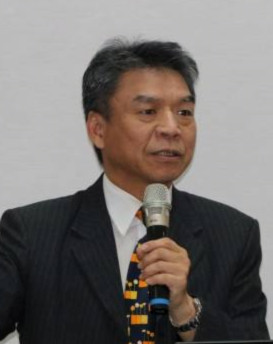
|
|
Title: A Computer-aided System using Artificial Intelligence for Clinical Bone Age Estimation
Abstract:
A deep learning based automatic bone age identification system (ABAIs) was introduced in medical imaging. This ABAIs enhanced accurate, consistent, and timely clinical diagnostics and enlightened research fields of deep learning and artificial intelligence (AI) in medical imaging. The goal of this study was to use Deep Neural Networks (DNN) model of machine learning (ML) techniques to establish an algorithm or model to assess bone (skeletal) age in months based on a database of pediatric left-hand radiographs. The Inception Resnet V2 model with a Global Average Pooling layer to connect to a single fully connected layer with one neuron using the Rectified Linear Unit (ReLU) activation function consisted of DNN model for BAA in this study. The medical data in each case contained posterior view X-ray image of left-hand, age, gender, weight and clinical skeletal bone assessment. Cases of ages between 0 and 20 years old with their clinical records were selected and grouped by sex. A database consisting of 9,717 hand radiographs (8,867 training set and 850 testing set) with their gender and age (1-18 years) as the reference standard were used. The DNN model’s accuracies on the testing set were 77.4%, 95.3% and 99.7% within 0.5, 1 and 2 years of the ground truth respectively. The Mean absolute error for the study subjects was 0.33 and 0.25 year for male and female models, respectively. The ABAIs only took 4 ms to complete the analysis for each case. This system was very helpful for clinical teaching. It also helped to improve the accuracy and efficiency of clinical bone age assessment, and greatly reduced the burden on clinical personnel. Further, this system is stimulating the development of more AI tools and methods for other medical diagnostics.
Bio: Fuu-Jen Tsai is the vice President and Distinguished Professor of Pediatrics, China Medical University. He graduated from the China Medical University, Taichung, Taiwan and obtained his postdoctoral at the Institute of Chinese Medicine, China Medical University.
He became the Chief, Department of Medical Genetics at China Medical University in 1998. When he became Professor in Pediatrics in 2001, he was given an additional role as Chief, Department of Pediatrics from 2001 till 2003. Prof Tsai is currently the Chief, Department of Medical Research and Medical Genetics, China Medical University Hospital.
In addition, his academic responsibilities in China Medical University includes being the Dean of College of Chinese Medicine from 2003 to 2009, before assuming a new role as Dean of Office of Research and Development till 2015. He was promoted as Vice President in 2013 and appointed as Distinguished Professor of Pediatrics in 2015.
|
华刚 (便利蜂副总裁暨首席科学家)
|
|
Title: Towards Edge AI: Learning Quantization for Highly Accurate and Compact DNNs
Abstract:
Weight quantization is an effective way of compressing DNNs. It has also demonstrated to be very effective in speeding up the inference of DNNs, e.g., by replacing the heavy floating-point convolution operations with efficient bit-count operations. Despite the fact that the recognition accuracy has been continually improving on DNNs with quantized weights, there is still a significant gap in prediction accuracy when compared with full precision DNNs. We conducted a careful analysis of existing work in terms of training strategy, regularization, activation approximation, and quantization schemes. We were able to establish deep insights, and hence propose several principles and schemes, including a learning quantization scheme of the weights, to close this accuracy gap. The take home messages from my talk will be a set of secrete recipes to learn highly accurate and compact DNNs, which were extensively validated in large scale visual recognition tasks.
Bio:
Gang Hua is the Vice President and Chief Scientist of Wormpex AI Research. His research focuses on computer vision, pattern recognition, machine learning, robotics, towards general Artificial Intelligence, with primary applications in cloud and edge intelligence, and currently with a focus on new retail intelligence. Before that, he served in various roles at Microsoft (2015-18) as the Science/Technical Adviser to the CVP of the Computer Vision Group, Director of Computer Vision Science Team in Redmond and Taipei ATL, and Principal Researcher/Research Manager at Microsoft Research.
He was an Associate Professor at Stevens Institute of Technology (2011-15). During 2014-15, he took an on leave and worked on the Amazon-Go project. He was an Visiting Researcher (2011-14) and a Research Staff Member (2010-11) at IBM Research T. J. Watson Center, a Senior Researcher (2009-10) at Nokia Research Center Hollywood, and a Scientist (2006-09) at Microsoft Live labs Research. He received his Ph.D. degree in ECE from Northwestern University in 2006.
He is an IEEE Fellow, an IAPR Fellow, and an ACM Distinguished Scientist. He is the receipient of the 2015 IAPR Young Biometrics Investigator Award. He has published more than 150 peer reviewed papers in top conferences and journals. To date, he holds 19 US patents and has 15 more patents pending. He is a program chair for CVPR'2019 and CVPR'2022. He also serves as an Associate Editor in Chief for Computer Vision and Image Understanding, and an Associate Editor for the International Journal of Computer Vision.
|
原田达也
(东京大学 / 理化学研究所 RIKEN)
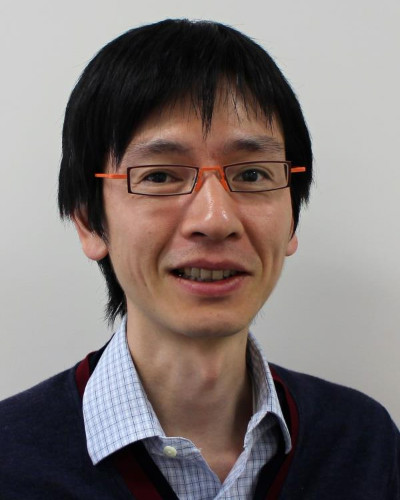
|
|
Title: Learning Deep Neural Networks from Limited Data
Abstract:
Training deep neural networks from limited data for constructing an accurate prediction model is one of the crucial tasks in machine learning. In this talk, we introduce unsupervised domain adaptation and learning method using between-class examples as a method to train DNNs from limited supervised data. Besides, we will briefly introduce various topics that we are working on in our team.
Bio: Tatsuya Harada is a Professor in the Department of Information Science and Technology at the University of Tokyo. His research interests center on visual recognition, machine learning, and intelligent robot. He received his Ph.D. from the University of Tokyo in 2001. He is also a team leader at RIKEN AIP and a vice director of Research Center for Medical Bigdata at National Institute of Informatics, Japan.
|
刘康 (中国科学院自动化所)
|
|
Title: Open Domain Knowledge Extraction
Abstract:
知识图谱是人工智能和核心基础设施之一,对于语义内容理解有重要的支撑作用。本报告结合研究组近些年的工作,主要介绍从非结构化文本中抽取结构化知识的基本方法,特别介绍在开放域环境下,面对多种知识类型,在缺乏标注数据的前提下,如何自动进行数据标注,训练鲁棒的知识抽取器的有效方法。
Bio: Kang Liu is an associate professor in Institute of Automation, Chinese Academy of Sciences. He also is a visting professor in Xidian University. His research areas include Information Extraction, Web Mining, Question Answering and Machine Learning. He has published more than 60 papers on top tier conferences and journals. He served as publicity cochairs for ACL-2015, area cochairs for ACL-2017 and ACL-2019. He won 2nd place in KDD CUP 2011 Track2, COL-ING 2014 Best Paper Award, “CCF-Tencent Xiniuniao” Excellence Award (The top award) in 2014, Hanwang Youth Innovation Excellence Award by Chinese Information Processing Society of China in 2014, and Google Focused Research Award in 2015,2016. He also won the first prize of "QianWeiChang Chinese Information Processing Science and Technology Award" of the Chinese Information Society in 2018.
|
林倞 (中山大学教授 / 暗物智能首席执行官)
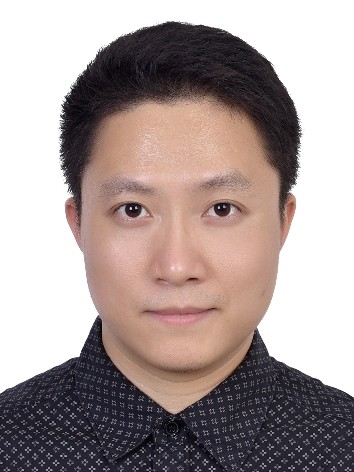
|
|
Title: Representation Learning Meets Knowledge Reasoning: New Trends in AI
Abstract:
With the development of deep representation learning methods, great progress has been made in many traditional perceptual AI tasks (e.g., face detection and speech recognition). These methods usually make prediction (e.g., classification or regression) by abstracting feature representations directly from the input data and however suffer from several problems such as the requirement of large amount of training data and the incapability on handling many higher-level applications (e.g., task planning and situational dialogue). Recently, much attention has been attracted for incorporating symbolic knowledge and commonsense inference with the feature representation learning, and a few pioneering works have demonstrated very promising results on harnessing the above-mentioned problems, which is the focus of this talk. And I will also introduce several relevant works on this new direction from my group, such as the graph reasoning for improving large-scale object detection and knowledge-routed relational dialogue system.
Bio: Liang Lin is the Professor of Sun Yat-sen University and DMAI CEO. He is the Excellent Young Scientist of the National Natural Science Foundation of China. He received his B.S. and Ph.D. degrees from the Beijing Institute of Technology (BIT), Beijing, China, in 2003 and 2008, respectively, and was a joint Ph.D. student with the Department of Statistics, University of California, Los Angeles (UCLA). From 2008 to 2010, he was a Post-Doctoral Fellow at UCLA. From 2014 to 2015, as a senior visiting scholar he was with The Hong Kong Polytechnic University and The Chinese University of Hong Kong. He currently leads the DMAI Inc. He has authorized and co-authorized on more than 100 papers in top-tier academic journals and conferences (e.g., 10 papers in TPAMI/IJCV and 30+ papers in CVPR/ICCV/NIPS/IJCAI). He has been serving as an associate editor of IEEE Trans. Human-Machine Systems, The Visual Computer and Neurocomputing. He served as Area/Session Chairs for numerous conferences such as ICME, ACCV, ICMR. He was the recipient of the Best Paper Runners-Up Award in ACM NPAR 2010, Google Faculty Award in 2012, and Best Student Paper Award in IEEE ICME 2014.
|
梁小丹 (暗物智能高级研究总监)
|
|
Title: Explainable AI with Deep Graph reasoning
Abstract:
Graph-structured representations are widely used as a natural and powerful way to encode information such as relations between objects or entities (e.g., in knowledge graphs), interactions between online users (e.g., in social networks), biological networks, 3D meshes in computer graphics, multi-agent environments, as well as molecular structure to name a few. How to efficiently model such data has been a long-standing research question of several areas, like machine learning, data mining and signal processing. Learning and reasoning with graph-structured representations is gaining increasing interest in both academia and industry, due to its fundamental advantages over more traditional unstructured methods in supporting interpretability, causality, transferability, etc. Graph-based learning and reasoning has found wide applications in drug discovery, scene graph generation, knowledge-based natural dialogue systems, conversational QA, interpretable and self-diagnosed medical analysis, recommendation and program synthesis. Recently, there is a surge of new techniques in the context of deep learning, such as graph neural networks, for learning graph representations and performing reasoning and prediction, which have achieved impressive progress. However, it can still be a long way to go to obtain satisfactory results in long-range multi-step reasoning, scalable learning with very large graphs, flexible modeling of graphs in combination with other dimensions such as temporal variation and other modalities such as language and vi-si-on. New advances in theoretical foundations, models and algorithms, as well as empirical discoveries and applications are therefore all highly desirable. This talk will overview the advances in graph neural networks and graph reasoning as well as the future direction.
Bio: Xiaodan Liang is currently a Senior Research Director at DMAI Group. She was a Project Scientist at Carnegie Mellon University, working with Prof. Eric Xing. She received her PhD degree from Sun Yat-sen University in 2016. She has published over 80 cutting-edge papers on graph neural networks, deep reasoning, structure prediction, object detection, which have appeared in the most prestigious journals and conferences in the field, Google Citation 3100+. She serves as an Area Chair of ICCV 2019. She and her collaborators has also published the largest human parsing dataset to advance the research on human understanding and successfully organized the 1st Look Into Person (LIP) and 2nd Look Into Person (LIP) workshop and challenge on CVPR 2017 and CVPR 2018. She hosts the “Towards Causal, Explainable and Universal Medical Visual Diagnosis Workshop” on CVPR 2019. She also hosted the tutorial about “Structured Deep Learning for Pixel-wise Understanding” on ACM MM 2018. She served as the guest editors of Pattern Recognition Letters and journal of Multimedia Tools and Applications. She obtained 2018 ACM China Best Doctoral Dissertation Award and CCF Best Doctoral Dissertation Award. She won the 2nd place in Key Points Detection of Apparel Track of FashionAI Global Challenge 2018-Alibaba Cloud.
|
申恒涛 (电子科技大学教授)
|
|
Title: Cross-media intelligence
Abstract:
It has been shown that heterogeneous multimedia data gathered from different sources in different media types can be often correlated and linked to the same knowledge space. Towards cross-media intelligence, cross-media understanding and retrieval has attracted huge amount of attention due to its significance in both research communities and industries. In this talk, we will introduce the state of the art on this topic and discuss its future trends.
Bio: Heng Tao Shen is currently a Professor of National "Thousand Talents Plan", the Dean of School of Computer Science and Engineering, the Executive Dean of AI Research Institute, and the Director of Centre for Future Media at the University of Electronic Science and Technology of China. He is also an Honorary Professor at the University of Queensland. He obtained his BSc with 1st class Honours and PhD from Department of Computer Science, National University of Singapore in 2000 and 2004 respectively. He then joined the University of Queensland as a Lecturer, Senior Lecturer, Reader, and became a Professor in late 2011. His research interests mainly include Multimedia Search, Computer Vision, Artificial Intelligence, and Big Data Management.
He has made continuous contributions to big multimedia data analysis, understanding, indexing and retrieval, and developed the first real-time near-duplicate video retrieval system. He has published 240+ peer-reviewed papers, including 2 survey articles “A survey on learning to hash” in IEEE TPAMI 2018 and “Near-duplicate Video Retrieval: Current Research and Future Trends” in ACM Computing Surveys 2013, 70+ IEEE/ACM Transactions, 170+ Chinese Computing Federation (CCF) A ranked papers in IEEE TIP, IEEE TPAMI, IEEE TNNLS, IEEE TKDE, ACM TOIS, VLDB Journal, ACM Multimedia, ACM SIGMOD, ACM SIGIR, VLDB, AAAI, IJCAI, CVPR, ICDE, etc. He has received 7 Best Paper Awards from international conferences, including the Best Paper Award from ACM Multimedia 2017 and Best Paper Award – Honorable Mention from ACM SIGIR 2017. Heng Tao received the Chris Wallace Award in 2010 conferred by Computing Research and Education Association, Australasia, the Future Fellowship from Australia Research Council in 2012, and the Overseas Chinese Outstanding Contribution Award of China in 2016.
Heng Tao is also active in community services. He is an Associate Editor of ACM Transactions of Data Science and IEEE Transactions on Knowledge and Data Engineering. He will organize ACM Multimedia 2021 as General Co-Chair in Chengdu China, and has organized ICDE 2013 as Local Organization Co-Chair, and ACM Multimedia 2015 as Technical Program Committee Co-Chair in Brisbane Australia.
|
吴大鹏 (佛罗里达大学教授)
|
|
Title: Tensor Product Generation Networks for Deep NLP Modeling
Abstract:
In this talk, I present a new approach to the design of deep networks for natural language processing (NLP), based on the general technique of Tensor Product Representations (TPRs) for encoding and processing symbol structures in distributed neural networks. A network architecture -- the Tensor Product Generation Network (TPGN) -- is proposed which is capable in principle of carrying out TPR computation, but which uses unconstrained deep learning to design its internal representations. Instantiated in a model for image-caption generation, TPGN out-performs LSTM baselines when evaluated on the COCO dataset. The TPR-capable structure enables interpretation of internal representations and operations, which prove to contain considerable grammatical content. Our caption-generation model can be interpreted as generating sequences of grammatical categories and retrieving words by their categories from a plan encoded as a distributed representation.
Bio: Dapeng Wu received Ph.D. in Electrical and Computer Engineering from Carnegie Mellon University, Pittsburgh, PA, in 2003. Since 2003, he has been on the faculty of Electrical and Computer Engineering Department at University of Florida, Gainesville, FL, where he is currently Professor. His research interests are in the areas of networking, communications, video coding, image processing, computer vision, signal processing, and machine learning.
He received University of Florida Term Professorship Award in 2017, University of Florida Re-search Foundation Professorship Award in 2009, AFOSR Young Investigator Program (YIP) Award in 2009, ONR Young Investigator Program (YIP) Award in 2008, NSF CAREER award in 2007, the IEEE Circuits and Systems for Video Technology (CSVT) Transactions Best Paper Award for Year 2001, the Best Paper Award in GLOBECOM 2011, and the Best Paper Award in QShine 2006.
Currently, he serves as Editor-in-Chief of IEEE Transactions on Network Science and Engineering. He was the founding Editor-in-Chief of Journal of Advances in Multimedia between 2006 and 2008, and an Associate Editor for IEEE Transactions on Communications, IEEE Transactions on Signal and Information Processing over Networks, IEEE Signal Processing Magazine, IEEE Transactions on Circuits and Systems for Video Technology, IEEE Transactions on Wireless Communications and IEEE Transactions on Vehicular Technology. He has served as Technical Program Committee (TPC) Chair for IEEE INFOCOM 2012. He was elected as a Distinguished Lecturer by IEEE Vehicular Technology Society in 2016. He is an IEEE Fellow.
|
俞益洲 (深睿医疗首席科学家)
|
|
Title: Image Recognition in Healthcare
Abstract:
In recent years, technological breakthroughs in the fields of deep learning and image recognition have triggered the rapid advancement of artificial intelligence in medicine and healthcare. The first part of the talk briefly introduces the relationship between healthcare and artificial intelligence as well as the characteristics of image recognition in the healthcare industry. The second part of the talk introduces the research achievements of Deepwise Healthcare in medical image recognition, including automatic diagnosis of pulmonary nodules in computed tomography, microcalcification detection in breast mammograms, and automated chest X-ray interpretation using mixed supervised learning.
Bio: Yizhou Yu is the chief scientist at Deepwise Healthcare. He was a tenured professor at University of Illinois, Urbana-Champaign (UIUC) for many years, and received his PhD degree in computer science from University of California, Berkeley. Professor Yu is an IEEE Fellow and ACM Distinguished Member. He has made important contributions to AI and visual computing. He is a recipient of US National Science Foundation CAREER Award, NNSF China Overseas Young Investigator Award, and ACCV Best Application Paper Award. His current research includes computer vision, deep learning, bioinformatics, medical image analysis, computational visual media, and geometric computing.
|
ACM SIGAI China 2019 征文:
本次论坛是ACM SIGAI中国的年度论坛,与中国图灵大会一起于2019年5月在成都举办,大会主题“注智世界,赋能未来”。论坛将邀请移动计算领域的专家学者、学生、业界精英共同探讨未来计算机应用技术领域的创新与产业机遇。
大会公开征稿,稿件形式为4-6页的论文。稿件正式截止日期为2019年3月1日。录用论文将收录于ACM Digital
library及EI。对于质量尤为突出的文章,还有机会进一步邀稿至四大AI相关的SCI期刊: Pattern Recognition, Multimedia Tools and Application, ACM Tomccap, IEEE Transactions on Image Processing。
欢迎大家将前沿探索或初步成果投稿至本次论坛。大家相聚成都!
The ACM Turing Celebration Conference - China (ACM TURC 2019), will be held at May 17-20, 2019,
Chengdu, Sichuan, with its key theme on "Interconnected, Inspired, intelligent (注智世界,赋能未来)".
The conference serves as a highly selective and premier international forum on computer science
research. In honor of Alan Turing, founder of computer logic and father of computer science, the
Association for Computing Machinery (ACM) established A. M. Turing Award in 1966 to award individuals
with remarkable contributions to the computer industry. The Turing Award is recognized as the highest
distinction in computer science and the Nobel Prize of computing.
For the past few years, the ACM Symposium on Artificial Intelligence has been a primary gathering forum
for promote and support the growth and application of AI principles and techniques throughout computing
around the world. ACM SIGAI CHINA symposium, co-located with ACM TURC 2019, aims to provide a world’s
premier forum of renowned researchers to share their insightful opinions, discuss the upcoming
challenges and promote the development of Artificial Intelligence.
We are seeking both innovative works in an unexplored and/or emerging topic in the broad area of
Artificial Intelligence, and novel findings and/or new insights built on existing works. We invite
submissions on a wide range of artificial intelligence research, including
but not limited to:
Artificial Intelligence
Computer Vision
Machine Learning
Deep Learning
Natural Language Processing
Speech Recognition
Speech synthesis
Question Answering
Virtual Reality
Cognitive Modeling
Data Analysis
Organization Committee
General Co-Chairs:
Liang Lin, Sun Yat-sen University
Wangmeng Zuo, Harbin Institute of Technology
Program Chairs:
Xiaodan Liang, Sun Yat-sen University
Yanli Ji, University of Electronic Science and Technology of China
Public Chairs:
Yan Gao, DMAI Inc.
Qilong Wang, Tianjin University
Submission Instructions
Papers should be submitted via https://easychair.org/conferences/?conf=acmturc2019
under SIGAI Track.
Submissions can either be two-page posters describing the novelties and highlights of the ongoing
work,or five-page double column papers. Papers must be submitted electronically in printable PDF form.
Templates for the standard ACM format can be found at https://www.acm.org/publications/proceedings-template.
No changes to margins, spacing, or font sizes are allowed from those specified by the style files.
Papers violating the formatting guidelines will be returned without review.
We adopt a double-blind review process for poster and regular papers. A short abstract must be provided
on the first page before the main body of the paper. For distinguishability, poster submission titles
must start with "Poster: ...". We particularly encourage and welcome contributions from students
submitting first results and early stage work.
Authors can also prepare full-version technical reports available on their own websites and on purely
archiving organizations such as arXiv. All accepted papers
will be entered into ACM database and the Engineering Index (EI). Accepted
papers with exceptional quality will further be invited into the fast track of SCI journals: Pattern Recognition, Multimedia Tools and Application, ACM Tomccap, IEEE Transactions on Image Processing.
Important Date
Paper Submission: 1st, March, 2019
Notification: 20th, March, 2019
Camera-ready: 31st, March, 2019