Symposiums--SIGAI China(Venue : Wanda Reign )
ACM SIGAI CHINA aims to carry out academic/technological exchanges on the professional contents of robotics, NLP, visual, big data, cognitive, brain science, and machine learning, and promote the integration of academia and industry closely and the industrial landing of AI technology. SIGAI CHINA will be carry out academic exchanges with ACM China to enhance the influence in national scientific and technological activities and international academic influence.
organizers
Chair
Yizhou Wang (Peking Unviersity)
Le Dong(Beijing Institute For General Artificial Intelligence)
Vice Chair
Gang Pan(Zhejiang University)
Yixin Zhu(Peking Unviersity)
Procedural Chairs
Quanshi Zhang(Shanghai Jiaotong University)
Ping Wei(Xi'an Jiaotong University)
Local Chair
Guisong Xia(Wuhan University)
Publicity Chairs
Guojie Song(Peking Unviersity)
Yifei Dong (Beijing Institute For General Artificial Intelligence)
Yanyun Qu(Xiamen University)
Industry Relationship Chairs
Wei Chen(Beijing Institute For General Artificial Intelligence)
Meeting schedule
Date(2023-07-29)Venue(TBC) | ||||
Time | Session | Title | Speaker | Host |
13:30-13:40 | Opening | Welcome Speech | Song-Chun Zhu, Director of the Beijing Institute for General Artificial Intelligence, Director of the Institute for Artificial Intelligence and School of Intelligence and Technology at Peking University, Chair Professor at Peking University and Tsinghua University | Le Dong, Deputy Director of the Beijing Institute for General Artificial Intelligence |
13:40-14:10 | Keynote | Human Rationality and Intelligence | Jun Zhang, Tenured Professor, at the Department of Psychology and the Department of Mathematics, University of Michigan | Yizhou Wang, Deputy Director of the Center on Frontiers of Computing Studies (CFCS) at Peking University, Boya Distinguished Professor |
14:10-14:40 | Keynote | Intelligent Information Processing - Insights from Brain Science | Wu Li, Professor and Director, State Key Laboratory of Cognitive Neuroscience and Learning, Beijing Normal University | |
14:40-15:10 | Keynote | Knowledge, reasoning and creativity in developing modern digital intelligence business technology – examples of PKU-Alimama Joint Laboratory for AI Innovation | Bo Zheng, CTO of Alimama & Xianyu, Chief Scientist of Alimama, executive member of CCF TCCE | |
15:10-15:30 | Teabreak | |||
15:30-15:50 | Invited Talk | Aligning Safe Decision-Making in Open Environments | Yaodong Yang, Assistant Professor and Doctoral Supervisor at the Institute for Artificial Intelligence, Peking University | |
15:50-16:10 | Invited Talk | Scene Understanding and Task Motion Planning for Robots | Hangxin Liu, Co-Chair of the Research Center at the Beijing Institute for General Artificial Intelligence, Head of the Robotics Laboratory | |
16:10-17:30 | Panel | TBD | Yizhou Wang (Host), Song-Chun Zhu, Jun Zhang, Wu Li, Bo Zheng, Yaodong Yang, Hangxin Liu |
Date(2023-07-30)Venue(TBC) | ||||
Time | Session | Title | Speaker | Host |
14:00-14:05 | Warm-Up | Warm-Up | Quanshi Zhang, School of Electronic Information and Electrical Engineering, Shanghai Jiao Tong University | |
14:05-14:25 | Invited Talk | Social Intelligence: Cognitive Modeling in Social Interaction | Lifeng Fan, Research Scientist at the Beijing Institute for General Artificial Intelligence | |
14:25-14:45 | Invited Talk | The Practice and Exploration of Conversational AI Technology | Yulong Wan, Director of the Department for Intelligent Interaction, Chief Architect of XiaoBu Assistant at OPPO | |
14:45-15:05 | Invited Talk | Causal Reasoning in Time-series data | Xinwei Sun, Assistant Professor at Fudan University | |
15:05-15:25 | Invited Talk | Can Graph Neural Networks Solve Practical Problems? | Di He, Assistant Professor and Doctoral Supervisor at Peking University | |
15:25-15:45 | Teabreak | Teabreak | - | Teabreak |
15:45-16:05 | Invited Talk | AI Music and Aesthetics | Xin Jin, Associate Professor at Beijing Electronic Science and Technology Institute, Director of Visual Computing and Security Laboratory (victory-lab) | Yanyun Qu, School of Computer Science and Technology, Xiamen Universitys |
16:05-16:25 | Invited Talk | Active Reasoning: Towards Unifying Knowing and Acting | Chi Zhang, Research Scientist at the Beijing Institute for General Artificial Intelligence | |
16:25-16:45 | Invited Talk | Value-driven Autonomous Agents | Chi Zhang, Research Scientist at the Beijing Institute for General Artificial Intelligence | |
16:45-17:05 | Ending | Ending Remark | Yizhou Wang, Deputy Director of the Center on Frontiers of Computing Studies (CFCS) at Peking University, Boya Distinguished Professor |
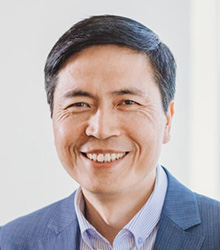
Invited Talk:

Song-Chun Zhu
Beijing Institute for General Artificial Intelligence
Chair Professor at Tsinghua Universitya nd
Peking University
Abstract:
BIO:Song Chun Zhu is a globally well-known Computer Vision scientist, Statistician and Applied Mamathematician, and artificial intelligence expert. In 1991, he earned his B.S. in Computer Science from the University of Science and Technology of China, and got his PhD from Harvard University in 1996. He has published more than 300 papers in top international journals and conferences, has won the Marr Prize, the top award in the Computer Vision field, three times, and has twice served as the general chair for the Conference on Computer Vision and Pattern Cognition (CVPR) in 2012 and 2019. Since 2010, he twice served as Principal Investigator for Multidisciplinary University Research Initiative (MURI) program, which supported research teams whose research efforts intersect the Vision, Cognitive Science and AI fields. In 2002, Zhu joined UCLA, served as a professor in the Department of Statistics and Computer Science, and became the Director of the UCLA Center for Vision, Cognition, Learning and Autonomy Director. In September 2020, he returned to China and established Beijing Institute for General Artificial Intelligence, serving as founding director. He was appointed the director of the School of Intelligence Science and Technology at Peking University and the Institute for Artificial Intelligence at Peking University.
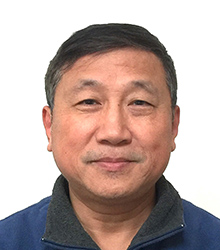
Invited Talk:Human Rationality and Intelligence

Jun Zhang
Tenured Professor
Department of Psychology and the Department of Statistics, University of Michigan
Abstract:My talk will discuss the role of rationality in human intelligence and general artificial intelligence (GAI). To illustrate rationality for reasoning, memory, and decision-making processes, the lecture will showcase various pitfalls and cognitive biases, and failures of the human mind. By studying the failure of rationality, the hope is to instigate the audience’s curiosity into the intertwining topics of mind and cognition, machine intelligence, and mathematical reasoning.
BIO:Jun Zhang is a fellow of the Association of Psychological Sciences and a fellow of the Psychonomic Society. He has served as the President, Vice President, and member of the Executive Board of the Society for Mathematical Psychology, and as a Council Member and elected Governing Board Member of the Federation of Associations in Brain and Behavioral Sciences (FABBS). He obtained his Ph.D. from the University of California at Berkeley in 1992. During sabbatical years, he held visiting positions at the University of Melbourne (Australia), CNRS Marseille (France), University of Waterloo (Canada), RIKEN Brain Science Institute (Japan), Newton Institute at Cambridge (UK), and Center for Mathematical Sciences and Applications (CMSA) at Harvard University. Jun Zhang directs the M3 Lab (“Mind, Machine and Mathematics”) which conducts research spanning cognitive science, machine learning, human-system interface, brain-like computation and artificial intelligence, under continuous grant support from US National Science Foundation (NSF) and the Department of Defense (DOD).
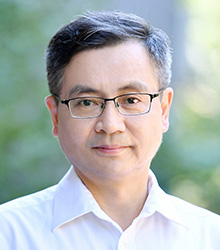
Invited Talk: Intelligent Information Processing—Insights from Brain Science

Wu Li
Professor and Director, State Key Laboratory of Cognitive Neuroscience and Learning, Beijing Normal University
Abstract: The advancements in artificial intelligence (AI) have benefited from breakthroughs in brain science, yet a substantial gap persists between the two fields. For instance, the brain's visual system, with its bottom-up hierarchical processing mode, has inspired the development of deep convolutional artificial neural networks; however, there remains limited convergence between the two, particularly in the principles governing information integration and readout that allow for perceptual flexibility. These principles involve intricate interactions between low- and high-level brain regions as well as functional systems beyond the visual system. Contemporary AI models frequently outperform the human brain in specific tasks by leveraging large-scale models, vast amounts of data, and powerful computational resources, rather than embodying the intricate workings of the brain. Nonetheless, the brain's intelligent information processing principles offer invaluable insights for overcoming the inherent limitations in existing AI algorithms. In turn, AI can provide valuable models for simulating and validating the brain’s inner workings, fostering mutual inspiration and iterative development between the two fields. This talk will discuss recent research findings related to the above topics.
BIO:Served as director of the IDG/McGovern Institute for Brain Research at Beijing Normal University (2013-2022). Recipient of a number of national awards and major grants; standing council member of the Chinese Neuroscience Society and chairman of the Cognitive Neuroscience Branch. Received the bachelor's degree in biophysics from University of Science and Technology of China (1989) ; obtained the PhD degree in neurobiology from Shanghai Institute of Physiology, Chinese Academy of Sciences (1994); postdoctoral researcher in psychophysics at the University of California, Berkeley (1994-1996); visiting scholar in Max Planck Institute for Biological Cybernetics, Germany (1996-1999); research assistant professor at Rockefeller University (1999-2006); joined Beijing Normal University in 2007. Research interests: Neural mechanisms of visual perception and visual learning; interactions between perceptual, cognitive and emotional processes in the brain.
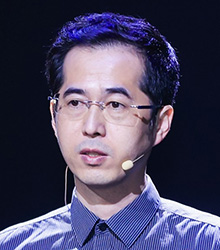
Invited Talk:Knowledge, reasoning and creativity in developing modern digital intelligence business technology – examples of PKU-Alimama Joint Laboratory for AI Innovation

Bo Zheng
CTO of Alimama & Xianyu
Chief Scientist of Alimama, executive member of CCF TCCE
Abstract: The Alimama technical team is committed to developing the most cutting-edge artificial intelligence technology and applying these technology in the business scenario, enabling more brands and small-and-medium-sized businesses to enjoy the benefits brought by technology development. In this wave of artificial intelligence technology represented by generative AI large model, we believe that the future-oriented AI business technology will have distinctive characteristics like knowledge-driven, logical reasoning and innovative. Inspired by this trend, the Alimama technical team has been conducting technical explorations for the application of such technology in fields such as generative AI large model. Meanwhile, we have carried out industry-academic cooperation with well-known universities and research institutions in China. For instance, since the official establishment of the PKU-Alimama Joint Laboratory for AI Innovation (PAAI) last year, we have carried out specific research and applications in the fields of large-scale graph models, decision intelligence, and intelligent music generation. This report will introduce our progress in both independent development and collaborative development in artificial intelligent technology.
BIO:In charge of Alimama & Xianyu’s algorithm, machine learning and engineering architecture. Bo Zheng was graduated from Department of Computer Science and Technology,Tsinghua University. Before joining Alibaba in 2017, he worked at Google for 11 years as a team leader leading Google’s global display advertising algorithm team and Google Maps team in China. Research orientations: deep learning, display/search advertising algorithm, multi-modal computing and computing engineering.
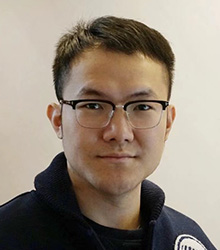
Invited Talk:Aligning Safe Decision-Making in Open Environments

Yaodong Yang
Assistant Professor and Doctoral Supervisor at the Institute for Artificial Intelligence, Peking
University
Researcher at the Beijing Institute for General Artificial Intelligence
2022 ACM SIGAI China Rising Star Award recipient
Abstract: Large language models exhibit impressive results in intelligent question-answering tasks. Utilizing the general knowledge within these models to aid intelligent decision-making is a key step toward achieving general artificial intelligence. In this presentation, I will introduce the process of decision model serialization and the alignment issues between decision models and large language models. Significant advancements in related technology have been achieved in areas such as dexterous hand manipulation and Minecraft.
BIO:Recipient of the Ministry of Human Resources and Social Affairs' High-Level Overseas Talent Return Program and the China Association for Science and Technology's Youth Support Plan Graduated from the University of Science and Technology of China with a Bachelor's degree; earned Master's and Doctoral degrees from Imperial College London and University College London. Prior to returning to China, served as an Assistant Professor at the School of Informatics, King's College London. Published over 60 articles and patents in top AI conferences and journals, receiving over 2000 citations on Google Scholar. Received the Conference on Robot Learning (CoRL) 2020 Best System Paper Award, the 2021 AAMAS Most Visionary Paper Award, and was recognized as a Bright Star at the World Artificial Intelligence Conference (WAIC 2022) for contributions to multi-agent reinforcement learning. Research areas include reinforcement learning, game theory, and multi-agent systems, with a focus on intelligent swarm game based on reinforcement learning methods and the emergence of collective intelligence.
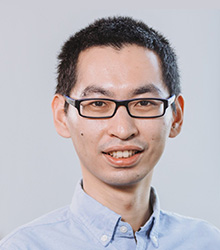
Invited Talk:Embodied Intelligence: Scene Understanding and Task Motion Planning for Robots

Hangxin Liu
Co-Chair of the Research Center at the Beijing Institute for General Artificial Intelligence
Head of the Robotics Laboratory
Abstract: This talk introduces a robot modeling approach based on virtual kinematic chains, which allows the robot's mobile base, robotic arm, and manipulated objects to be modeled as a whole. This comprehensive representation of the robot's posture and motion enables more coordinated and flexible actions in challenging environments. This modeling approach further drives a scene reconstruction method that aims to capture motion information. The method constructs a scene graph representation from RGB-D input that integrates with the virtual kinematic chain idea, realizing a new robot perception and planning framework. This framework demonstrates enhanced robot mobility, manipulation, and grasping capabilities in multi-step complex moving operation tasks.
BIO:Engaged in research on autonomous robot perception, planning, learning, and interaction, supported by funding from agencies such as the US Defense Advanced Research Projects Agency (DARPA) and the Office of Naval Research (ONR) (DARPA SIMPLEX, DARPA XAI, ONR MURI). Earned a Ph.D. in Computer Science and a Master's degree in Mechanical Engineering from the University of California, Los Angeles. Published nearly 30 papers in top journals and academic conferences in the fields of robotics, computer vision, and artificial intelligence, including Science Robotics, IJCV, Engineering, RA-L, ICRA, IROS, and AAAI. Received the Best Paper Award at the 2019 ACM Turing Conference in China.
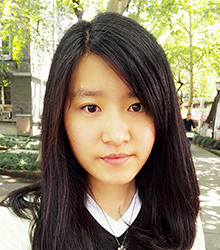
Invited Talk:Social Intelligence: Cognitive Modeling in Social Interaction

Lifeng Fan
Research scientist of the Cognitive and Reasoning Laboratory at the Beijing Institute for General Artificial Intelligence
Abstract: In addition to a physical comprehension of the world, humans possess a high social intelligence—the intelligence that senses social events infers the mental states of others, and facilitates social interaction. We believe that Artificial Social Intelligence (ASI) will play a crucial role in shaping the future of artificial intelligence (AI). We propose a new hierarchical computational framework for social interaction understanding and the belief dynamics modeling.
BIO:Obtain her Ph.D. degree from University of California, Los Angeles ( UCLA) under the supervision of Prof. Song-Chun Zhu. Research interests include cognitive modeling, nonverbal communication and social interaction modeling. Have published more than 10 papers in top international journals and conferences such as ICCV, CVPR, NeuRIPS, etc. Her work has been widely reported by the media. Recipient of the Best Modeling Prize from the International Cognitive Society, and the 2021 ACM SIGAI China Rising Star Award.
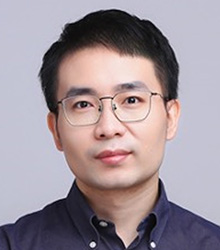
Invited Talk:The Practice and Exploration of Conversational AI Technology

Yulong Wan
OPPO Director of the Department for Intelligent Interaction
Chief Architect of XiaoBu Assistant
Abstract:With the rapid development of AIGC technologies like GPT and Diffusion, demand for conversational AI products is beginning to explode. Products such as Midjourney and ChatGPT are becoming popular, and more and more domestic and international enterprises are increasing their investments in conversational AI technology. As a team with hundreds of millions of monthly active users of our conversational AI assistant, we are actively thinking about and exploring the evolutionary path under this new AI wave. This presentation mainly discusses OPPO's technological evolution and product innovation in terms of conversational AI assistants, along with the discussion on future industry development trends.
BIO:Bachelor of Science and Bachelor of Economics from Peking University, PhD from the Chinese Academy of Sciences. Former Director of the Technical Committee for OPPO's Intelligent Assistant. Professional member of CCF, IEEE, and CAAI. Executive committee member of CCF's Human-Computer Interaction, Speech Dialogue & Auditory, and Virtual Reality. Member of the CCF CTO Club. Previously worked at the Institute of Acoustics of the Chinese Academy of Sciences and Alibaba DAMO Academy, engaging in long-term research and development as well as productization work in human-computer interaction technology such as voice semantics and multimodal interactions.
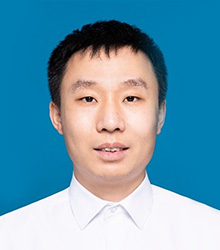
Invited Talk:Causal Reasoning in Time-series data

Xinwei Sun
Assistant Professor at Fudan University
2022 ACM SIGAI China Rising Star Award recipient
Abstract:Time series data is widely present in artificial intelligence applications, such as video analysis, robot planning, and brain neurosignal analysis, among others. Inferring causal relationships between events from time series data is significant for planning and decision-making. However, existing methods face the problem of unidentifiable causal graphs, leading to erroneous inference results. We first note that when the sampling frequency is lower than the frequency of causal influence, unobserved subsampling points are generated, which cause biased inference. To remove this bias, we treat the future observations of these subsampling points as proxy variables and transform the inference of causal relationships into a hypothesis testing problem using these proxy variables. We prove that this method can unbiasedly recover the causal graph. We apply the proposed approach to learn the causal chain of brain region propagation in Alzheimer's disease, and compared to existing methods, this chain exhibits stronger interpretability.
BIO:Received a Ph.D. from Peking University in 2018, served as a machine learning researcher at Microsoft Research Asia from 2019 to 2022. Joined the School of Big Data at Fudan University in March 2022. Published papers in journals such as Applied and Computational Harmonic Analysis, IEEE TPAMI, IEEE Transactions on Signal Processing, IEEE Transactions on Image Processing, as well as international machine learning conferences like ICML, NeurIPS, and ICLR. Research interests: High-dimensional statistics, causal inference theory, and their applications in the field of machine learning.
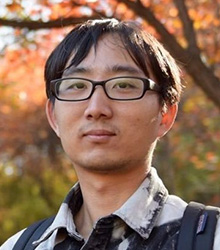
Invited Talk:Can Graph Neural Networks Solve Practical Problems?

Di He
Assistant Professor at Peking University
Abstract:Designing expressive Graph Neural Networks(GNNs) is a central topic in learning graph-structured data. While numerous approaches have been proposed to improve GNNs with respect to the Weisfeiler-Lehman (WL) test, for most of them, there is still a lack of deep understanding of what additional power they can systematically and provably gain. In this work, we take a fundamentally different perspective to study the expressive power of GNNs beyond the WL test. Specifically, we introduce a novel class of expressivity metrics vla graph biconnectivty and highlight their importance in both theory and practice. We introduce a principled and efficient approach called the Generalized Distance Weisfeiler-Lehman (GD-WL), which is provably expressive for all biconnectivity metrics. Practically, we show GD-WL can be implemented by a Transformer-like architecture that preserves expressiveness and enjoys full parallelizability.
BIO:Published dozens of papers at top machine learning conferences, including ICML, NeurIPS, and ICLR. Served as an area chair and reviewer for top machine learning conferences. Won several awards in the competitions, including the first place of the Molecular Property Prediction Challenge at KDD 2021 and the Molecular Dynamics Simulation Challenge at NeurIPS 2021. Received the ICLR 2023 Outstanding Paper Award.
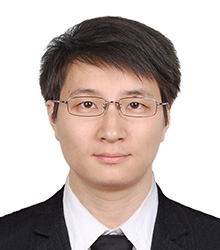
Invited Talk:AI Music and Aesthetics

Xin Jin
Associate Professor at Beijing Electronic Science and Technology Institute
Director of Visual Computing and Security Laboratory (victory-lab)
Co-Head of AI Music Project at Beijing Institute for General Artificial Intelligence
Abstract:Artificial intelligence music generation is one of the hot research topics in the AIGC field. Current methods are mostly based on language-related deep learning models that imitate and generate from human-made music data. However, there are still significant shortcomings in interpretability, controllability, and interactivity. There is a lack of professional music annotation datasets, and less consideration is given to musical aesthetics and alignment with human values. We propose a vertical-horizontal research approach in the field of AI music. Vertically, it introduces a Music And-Or Graph (Music AOG) representation. Horizontally, it investigates composition, arrangement, and audio generation based on Music AOG. The goal is to achieve node-by-node and process-by-process interpretability, controllability, and interactivity in both vertical music structure and horizontal music production. This talk mainly covers explorations in AOG representations for melody, chords, harmony, rhythm, the Music Parser professional music annotation tool, music sampling generation, music UV theory, computational music aesthetics, and video scoring applications.
BIO:Earned a Ph.D. in Computer Science from Beihang University under the guidance of Academician Zhao Qinping; visited Lotus Hill Computer Vision Research Institute under the supervision of Professor Zhu Songchun; and was a visiting scholar at Tsinghua University under the guidance of Academician Dai Qionghai and Professor Liu Yebin. Main research areas include computational aesthetics, computer vision, and AI security. Published over 70 papers in important academic journals and conferences in the fields of computer vision, image processing, artificial intelligence, and multimedia technology, including TIP, TMM, TITS, SC-IS, ACM MM, AAAI, IJCAI, CVPR, and ECCV. Holds 31 granted national invention patents, received the 2023 Golden Seagull Film & Television Honorary Outstanding Technical Contribution Award, the ROSENET 2018/19 Best Paper Award, and the ISAIR 2017 Best Student Paper Award. Participated in the formulation of the national standard "Information Technology - Computer Vision Terminology" by the China Communications Standards Association (CCSA). Led or participated in nine national-level research projects and more than 20 provincial and enterprise cooperation projects (including Huawei, Alibaba, China Ordnance Industry, and Sinopec). Senior member of CCF, serving as executive committee member of ISAIR, CSIG Visual Big Data Committee, deputy secretary-general of CIE Virtual Reality Branch, executive member of CCF Computer Vision Committee, Computational Art Branch, and member of China Film Art Association Virtual Space Committee.
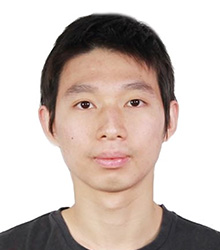
Invited Talk:Active Reasoning: Towards Unifying Knowing and Acting

Chi Zhang
Research Scientist at the Beijing Institute for General Artificial Intelligence
Abstract: Recent advances in language and vision-language learning have shown startling results on a range of complete-information question-answering datasets by incorporating vast amounts of world knowledge into the learned model. Instead of merely being passively questioned and retrieving from a knowledge base, humans can actively respond, explore, gather, and reason from both the new and the known to derive a solution to incomplete-information questions. To fill in this paradoxically absent but critical gap, we introduce a suite of reasoning problems in interactive environments, to motivate and evaluate agents for this active reasoning ability. Specifically, the suite of tasks affords active exploration and multi-round inference for questions with incomplete information in knowledge-rich environments. The suite of tasks cover intuitive physics, causal induction, and abductive reasoning. Comparably, existing works for embodied reasoning only task agents to perform single-round deduction realizable through instruction following, ignoring the active nature of human reasoning. In comparison, in the suite of tasks, agents are required to actively interact with the environment to both seek out fresh evidence and combine existing knowledge to explain what occurred based on observation. We examine different multiple state-of-the-art models, showing their limits in actively exploring the environment and comprehending complex scenarios. We hope the suite of tasks would motivate, evaluate, and improve active reasoning agents and pave the way for future artificial intelligence agents that unify knowing and acting.
BIO:Chi Zhang was supervised by Prof. Song-Chun Zhu at UCLA and a member of the VCLA lab. His research focus is on neuro-symbolic visual reasoning, ranging from visual-numerical reasoning, to spatial-temporal reasoning, to a unified theory of general abstract reasoning, with technique involving probabilistic inverse/forward planning and bilevel optimization. His research also involves emerging topics on style transfer, causal reasoning, physical reasoning, abductive reasoning, and probing language models. Chi has authored and co-authored several papers in IJCAI, AAAI, CVPR, ECCV, NeurIPS, IROS, and ICRA. Chi was once among the top developers for MXNet. During his PhD, Chi served as a research intern at Amazon studying active domain adaptation, at Google for efficient Transformer Detector, and at Beijing Institute for General Artifical Intelligence for the unified theory. Before his PhD, Chi got a Master's degree from UCLA and a Bachelor's degree from Zhejiang University.
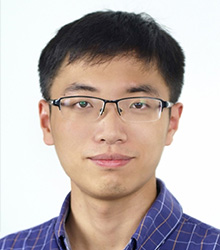
Invited Talk:Value-driven Autonomous Agents

Fangwei Zhong
Boya Postdoctoral Fellow at Peking University
Recipient of the 2021 ACM SIGAI China Doctoral Dissertation Award
Abstract:Autonomous agents are computational systems that can actively perceive and act in complex and dynamic environments, pursuing a set of self-motivated goals or tasks. In this talk, I will present our research on developing general value systems that can effectively drive agents to accomplish various tasks. The generality of the value system will be closely related to the generality of the autonomous agents. Specifically, I will discuss three main ways to construct value systems: prior knowledge, human feedback, and Interactive use. I will also demonstrate how to use the value system to guide the agent's decision making in open-ended tasks and multi-agent settings.values.
BIO:Published over 20 high-quality papers in top international journals and conferences in the field of artificial intelligence, including 2 IEEE TPAMI articles (IF 17.86, SCI Q1) and conference papers for ICLR, ICML, NeurIPS, CVPR, etc. Served as a reviewer for top-tier journals and conferences such as Nature Machine Intelligence and International Journal of Robotics Research (IJRR). Funded by the National Postdoctoral Program for Innovative Talents ("BoXin" Plan) Core team member of the open-source project UnrealCV, providing near-realistic virtual environments for AI research. Research interests: Autonomous learning, multi-agent systems, cognitive reasoning