Symposiums--SIGBED China(Venue : Wanda Realm )
The ACM Special Interest Group on Embedded Systems China Chapter (ACM SIGBED China Chapter) is a professional committee mainly aimed at the field of embedded systems, providing a platform for experts, scholars, and industrial R&D personnel in the fields of embedded systems, Internet of Things, electronic design automation, and related areas. The committee is committed to promoting advanced embedded technology to various application fields and promoting industry-academia-research cooperation. The fields of communication include, but are not limited to: embedded software and hardware, embedded system design, Internet of Things, hybrid information physical systems, embedded artificial intelligence, edge computing, intelligent systems and related theoretical and application fields.
Organization Committee
General Co-Chairs:
Jie Xiong (MSRA)
Yunxin Liu (Tsinghua AIR)
Program Committee Chairs:
Mengwei Xu (BUPT)
Weixi Gu (CAII)
Meeting schedule
Date(2023-07-29) Location(多功能厅1+2) | |||||
Time | Talks | Moderator | Speaker | ||
14:00-14:05 | Opening Remarks | Yunxin Liu, Institute for AI Industry Research, Tsinghua University | Jie Liu, Harbin Institute of Technology | ||
14:05-14:45 | Keynote 1 | Internet of Medical Things: Precision Medicine with AI and Wearables | Chenyang Lu, Washington University in St.Louis | ||
14:45-15:25 | Keynote 2 | Digital Twin System for the Industrial Park: Research and Exploration of Integrated Perception of All Elements of Transportation | Yanyong Zhang, University of Science and Technology of China | ||
15:25 - 15:40 | 茶歇 | ||||
15:20 - 15:50 | Keynote 3 | Infrastructure-assisted Autonomous Driving Systems | Ting Cao, MSRA | Guoliang Xing, The Chinese University of Hong Kong | |
16:20 - 17:20 | Panel | Opportunities and Challenges in Constructing IoT Foundation Model | Chenyang Lu, Yanyong Zhang, Guoliang Xing, Lili Qiu, Zhijun Li |
Date(2023-07-30) Location(多功能厅1+2) | |||||
Time | Talks | Moderator | Speaker | ||
14:00-14:05 | Opening Remarks | Mengwei Xu, Beijing University of Posts and Telecommunications | - | ||
14:05-14:35 | Invited Talk 1 | Towards in-network Control-as-a-Service for real-time industrial networks | Zheng Yang, Tsinghua University | ||
14:35-15:05 | Invited Talk 2 | LUT-NN: Empower Efficient Neural Network Inference with Centroid Learning and Table Lookup | Ting Cao, MSRA | ||
15:05-15:35 | Invited Talk 3 | Towards Efficient and Scalable Edge Video Analytics | Yuanchao Shu, Zhejiang University | ||
15:35 - 15:50 | Teabreak | ||||
15:50 - 16:20 | Invited Talk 4 | Advancing Wearable Sensing: Integration of the Physical and Cyber Worlds | Yuanchun Li, Institute for AI Industry Research, Tsinghua University | Zhenyu Yan, The Chinese University of Hong Kong | |
16:20 - 16:50 | Invited Talk 5 | Millimeter-scale Movement Tracking with Magnetic Sensing | Dongyao Chen, Shanghai Jiao Tong University | ||
16:50 - 17:20 | Invited Talk 6 | Carbon emissions and sustainability of 5G mobile networks in China | Tong Li, Tsinghua University |
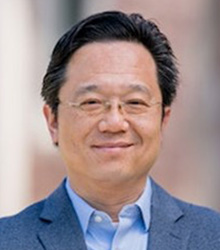
Topic: Personalized Predictions of Clinical Outcomes and Treatment Response with Wearables and Machine Learning

Chenyang Lu
Washington University in St. Louis
Fullgraf Professor
ACM/IEEE Fellow, Director, AIM
Institute
the Editor-in-Chief of ACM Transactions on Cyber-Physical Systems
Abstract: :Driven by growing adoption of wearable devices and advancements in artificial intelligence, Internet of Medical Things (IoMT) have emerged as new clinical instruments for precision medicine. Wearable devices enable unobtrusive monitoring of patients in their daily lives. To realize their potential in precision medicine, we need to develop machine learning models capable of extracting reliable clinical information from noisy and incomplete wearable data. Furthermore, the ML approaches need to scale effectively across a wide range of sample sizes. They should provide robust predictions in the presence of moderate amounts of data, while improving predictive power when large amounts of data become available. Finally, to support precision medicine, ML models need to make personalized prediction of not only the clinical outcomes of a patient but also their response to a specific treatment so that the treatment can be tailored for the patient. This talk will present our recent efforts to tackle these challenges in three clinical studies using Fitbit wristbands. 1) We built a robust feature engineering and ML pipeline tailored for wearable studies with limited sample sizes. We demonstrated the effectiveness of the pipeline in predicting post-operative complications and hospital readmissions in a prospective clinical trial of patients undergoing pancreatic surgery. 2) We developed WearNet, an end-to-end deep learning model for detecting mental disorders using Fitbit data. WearNet effectively enhanced predictive performance by exploiting a large public dataset including 8,996 participants and 1,247 diagnosed with mental disorders. 3) We explored multi-task ML to predict individualized treatment response to depression therapy based on wearable data collected from a randomized controlled trial (RCT) for patients undergoing behavioral therapy. Results from the clinical studies demonstrated the effectiveness of ML in predicting clinical outcomes in the presence of noise and missing data collected by wearables. Furthermore, ML provides a promising approach to predict individualized treatment response by leveraging data collected in RCTs. We will conclude the talk by highlighting the opportunities and directions in advancing IoMT as vital instruments for precision medicine.
BIO:Chenyang Lu is the Fullgraf Professor of Computer Science and Engineering and Professor of Medicine at Washington University in St. Louis. His research interests include artificial intelligence (AI) for medicine, Internet of Things (IoT), real-time systems, and cyber-physical systems. He is the founding director of the AIM Institute, a cross-school, interdisciplinary research hub dedicated to AI and IoT for medicine. He received the Outstanding Technical Achievement Award and Leadership Award from IEEE Technical Community on Real-Time Systems (TCRTS). He was also recognized by a SenSys Test-of-Time Award, an RTAS Influential Paper Award, and eight Best and Outstanding Paper Awards. He is currently Editor-in-Chief of ACM Transactions on Cyber-Physical Systems. He also served as Editor-in-Chief of ACM Transactions on Sensor Networks and Chair of TCRTS. He is a Fellow of ACM and IEEE.
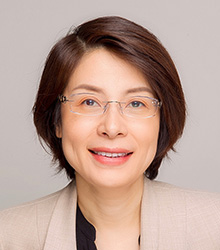
Topic: Digital Twin System for the Industrial Park: Research and Exploration of Integrated Perception of All Elements of Transportation.

Yanyong Zhang
Professor and associate dean, School of Computer Science at USTC
Vice Chairman of ACM
China
IEEE Fellow
Abstract:Digital twin can endow physical entities with identity and information interaction capabilities. To construct a digital twin system through integrated perception of all roadside traffic elements, various challenges need to be addressed, such as cumbersome sensor calibration, insufficient perception accuracy, and ineffective utilization of data. In this regard, we propose to utilize the natural features in the road environment and vehicle trajectory data to achieve online sensor calibration. Additionally, we employ pure visual perception and multimodal Bird's Eye View (BEV) fusion perception technology to effectively perceive traffic elements. Furthermore, by leveraging data characteristics, we establish data models and spatiotemporal indices for efficient storage and querying of perception data. This talk focuses on our research and exploration in the area of digital twin systems for integrated perception of all roadside traffic elements in a park.
BIO:Yanyong Zhang is a full professor and associate dean of School of Computer Science at USTC. She is the vice president of ACM China Council, chief scientist of National Key Research and Development Program of China, and an IEEE Fellow. She was a full professor, associate professor, and assistant professor of Rutgers University. She has served on many organization committees and TPC committees of international conferences, including TPC co-chair for ACM/IEEE IPSN 2022. She has served as the Associate Editor for the following journals: IEEE TCC, IEEE TDSC, IEEE/ACM ToN, IEEE TMC, IEEE TSC, and Elsevier Smart Health. She is the winner of ACM MobiCom Best Paper Runner-up Award in 2021.
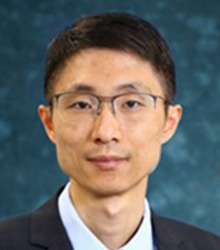
Topic: Infrastructure-assisted Autonomous Driving Systems

Guoliang Xing
Professor at The Chinese University of Hong Kong
IEEE Fellow
Abstract:Autonomous driving will revolutionize the future transportation systems. However, recent pilot commercial deployments have caused widespread concerns about the safety of emerging autonomous driving systems. I will discuss our recent work on leveraging intelligent roadside infrastructure to improve the safety of autonomous driving. First, we have deployed the first open smart lamppost testbed on CUHK campus which offers various real-time services such as target detection and dynamic route planning for vehicles. Second, we propose a novel real-time deep learning task framework, which integrate model architecture optimization and real-time scheduling to enable concurrent execution of multiple deep learning tasks. Third, I will present two new systems for real-time 3D perception fusion between vehicle and infrastructure with centimeter accuracy.
BIO:Professor Guoliang Xing is currently a professor in the Department of Information Engineering at The Chinese University of Hong Kong. He is an IEEE Fellow and has previously served as a tenured professor at Michigan State University in the United States. Professor Xing's research focuses on embedded artificial intelligence, autonomous driving, intelligent health, and the Internet of Things (IoT). His work has received over 11,000 citations. In 2010, Professor Xing received the National Science Foundation's CAREER Award, and in 2014, he was awarded the Distinguished Faculty Award at Michigan State University. His work has received Best Paper Awards and Best Paper Nomination at several top international conferences, including MobiCom, IPSN, ICNP, SenSys, and IoTDI.
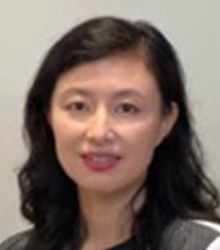

Lili Qiu
Assistant Managing Director, MSRA
ACM/IEEE Fellow
Chair of ACM SIGMOBILE
Bio:Dr. Lili Qiu is an assistant managing director of Microsoft Research Asia and is mainly responsible for overseeing the research, as well as the collaboration with industries, universities, and research institutes, at Microsoft Research Asia (Shanghai). Dr. Qiu is a leader in the fields of Internet, wireless networking, and sensing. She got PhD in Computer Science from Cornell University in 2001. She began her career at Microsoft Research Redmond, working as a researcher in the System & Networking Group from 2001 to 2004. In 2005, she joined the University of Texas at Austin as an assistant professor in the Department of Computer Science. Recognized for her outstanding achievements, she was later promoted to a tenured full professor. Her significant achievements have earned her several prestigious honors, including National Academy of Inventors (NAI) Fellow, ACM Fellow, and IEEE Fellow. Additionally, she serves as the chair of ACM SIGMOBILE. She has been recognized as an ACM Distinguished Scientist and received the NSF CAREER award, among many other honors.
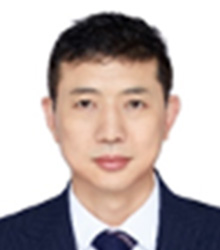

Zhijun Li
Professor at harbin institute of technology
BIO:Zhijun Li is a full professor at harbin institute of technology, chief scientist of leading IT Enterprises, deputy director of key laboratory of IoT intelligent technology, Ministry of Industry and Information Technology. He also serves as distinguished professor at Suzhou University, adjunct professor at Xiamen University, and member of ACM SIGBED China and CCF TCIoT. He has published more than 60 papers on top conferences/journals like IEEE/ACM TON、TMC、MOBICOM、MOBISYS、SENSYS、INFOCOM. His first-authored paper has won MobiCom Best Paper Award.
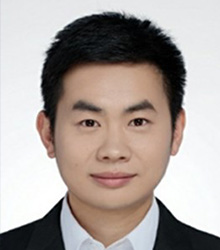
Topic: Towards in-network Control-as-a-Service for real-time industrial networks

Zheng Yang
Associate Professor at Tsinghua University
IEEE Fellow
Abstract: Flexible manufacturing presents new challenges for industrial control networks, such as the cumbersome configuration process, limited scalability, and high upgrade costs during production task switching. The fundamental reason is that control tasks are tightly coupled with controllers while regardless of network transmission. This project proposes a real-time industrial network architecture that supports Control as a Service (CaaS). CaaS technology decouples control tasks from dedicated controllers and deploys them flexibly in any switch within the network, based on coordinated scheduling of control tasks and network traffic. It virtualizes the entire industrial control network into a universal controller, achieving control function virtualization. Building upon this, the project employs a co-design approach of software and hardware to develop CaaS-enabled network switches and protocol stacks. A series of mechanisms are designed to ensure network determinism, computation determinism, and software-hardware data interaction determinism, ultimately guaranteeing end-to-end determinism of industrial control tasks from input to output. Experimental results demonstrate zero packet loss in CaaS, with a 42-45% reduction in latency and a three-order-of-magnitude reduction in jitter. We believe that CaaS will drive industrial control networks towards decentralization, virtualization, and service-oriented directions.
Bio:Zheng Yang is an Associate Professor and doctoral supervisor at Tsinghua University. He is also an IEEE Fellow. His research focuses on the Internet of Things (IoT) and Industrial Internet. Professor Zheng Yang has been honored with the Second Prize of the National Natural Science Award and has been selected for the National Youth Top Talent Support Program and the Beijing Nova Program. He has also received the National Science Fund for Excellent Young Scholars.
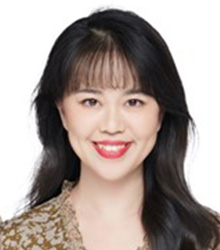
Topic: LUT-NN: Empower Efficient Neural Network Inference with Centroid Learning and Table Lookup

Ting Cao
Principal Researcher/Research Manager, MSR
Abstract:On-device Deep Neural Network (DNN) inference consumes significant computing resources and development efforts. To alleviate that, we propose LUT-NN, the first system to empower inference by table lookup, to replace the linear computation operators, and reduce inference cost. LUT-NN learns the typical features for each operator, named centroid, and precompute the results for these centroids to save in lookup tables. During inference, the results of the closest centroids can be read directly from the table, as the approximated outputs without computations. LUT-NN integrates two major novel techniques: (1) differentiable centroid learning through backpropagation, which adapts three levels of approximation to minimize the accuracy impact by centroids; (2) table lookup inference execution, which comprehensively considers different levels of parallelism, memory access reduction, and dedicated hardware units for optimal performance. LUT-NN is evaluated on multiple complex real tasks, covering image recognition, speech recognition, and nature language processing. Compared to related table lookup techniques for matrix multiplication, LUT-NN achieves similar-level of accuracy with the original models, and reduces the cost at all dimensions, including FLOPs (≤ 16×), model size (≤ 7×), latency (≤ 6.8×), memory (≤ 6.5×), and power (≤ 41.7%).
个BIO:Dr. Ting Cao is currently a Principal Researcher/Research Manager in the Heterogeneous Extreme Computing Group (HEX) at Microsoft Research. Her research focuses on deep learning systems, software-hardware co-design, high-level language implementation, and energy-efficient software-hardware design. She received her Ph.D. from the Australian National University's Research School of Computer Science. Prior to joining Microsoft, she worked at Huawei Compiler and Programming Language Laboratory, where she was responsible for the memory management system in the Ark Compiler. Her papers have been published in top conferences or journals in the field of systems, including MobiCom, MobiSys, ISCA, ASPLOS, and PLDI, among others. She has served on the program committees of conferences such as PLDI, OOPSLA, and MobiSys. She has received several awards, including the Best Paper awards at ICCD, NAS, and MobiSys, as well as ACM Research Highlights, IEEE Micro Top Picks, ACM SIGMOBILE Research Highlights, and the Huawei Star of Tomorrow Award.
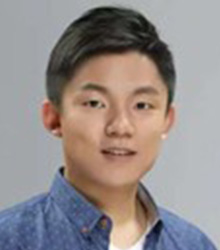
Topic: Towards Efficient and Scalable Edge Video Analytics

Yuanchao Shu
Qiushi Professor, Zhejiang University
Abstract:We are all living in the golden era of AI that is being fueled by game-changing systemic infrastructure advancements. Among numerous applications, video analytics, in particular, has shown tremendous potential to impact science and society due to breakthroughs in ML, copious training data, and pervasive deployment of video capture devices. Analyzing live video streams is arguably the most challenging of domains for “systems-for-AI”. Live video analytics require high bandwidth, consume considerable compute cycles for processing, necessitate richer query semantics, and demand tight latency, security and privacy guarantees. The talk will touch up on various advances in edge video analytics systems including real-time and efficient inference over edge hierarchies, collaborative analytics on camera networks, and continuous learning of models.
BIO:Yuanchao Shu is currently a Qiushi Professor with the College of Control Science and Engineering at Zhejiang University, China. Prior to joining academia, he was a Principal Researcher with Microsoft Azure and Microsoft Research Redmond. His research interests lie broadly in mobile, sensing and networked systems. His previous research results have led to over 60 publications at top-tier peer-reviewed conferences and journals. Dr. Shu currently serves on the editorial board of IEEE Transactions of Wireless Communications, ACM Transactions on Sensor Networks, and was a member of the organizing committee and TPC of conferences including MobiCom, MobiSys, SenSys, SEC, Globecom, ICC etc. He won five Best Paper/Demo (Runner-Up) Awards, and was the recipient of ACM China Doctoral Dissertation Award (2/yr) and IBM PhD Fellowship. Dr. Shu received his Ph.D. from Zhejiang University, and was also a joint Ph.D. student in the EECS Department at the University of Michigan, Ann Arbor. Dr. Shu is a senior member of ACM and IEEE.
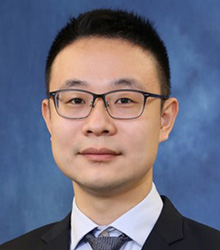
Topic: Advancing Wearable Sensing: Integration of the Physical and Cyber Worlds

Zhenyu Yan
Research Assistant Professor, The Chinese University of Hong Kong
Abstract:Wearable devices are at the forefront of ubiquitous mobile computing and sensing, profoundly shaping the interfaces between our physical and digital experiences. Enhanced by the leaps in AI and embedded computing technologies, we now witness the dawn of a new generation of wearable systems that harness multi-modal data for the creation of intelligent applications in ubiquitous environments. This talk will delve into a suite of innovative applications powered by wearable technologies, specifically focusing on their exploitation of bodily electrical potentials and acoustic signals.
BIO:Dr. Zhenyu Yan is a Research Assistant Professor at The Chinese University of Hong Kong. Dr. Yan has extensive experience in wearable sensing systems, signal and information processing, cyber-physical systems, and machine learning in IoT systems. His previous works have been published on premium international conferences and journals such as MobiCom, SenSys, IPSN, IEEE Transactions on Mobile Computing, and ACM Transactions on Sensor Networks. He is the recipient of Kan Tong Po International Fellowship from the Royal Society in the UK and “Rising Star Award” from ACM SIGBED China. His papers also receive “Best Paper Award Runner-up” at ACM MobiCom 2022 and “Best Artifact Award Runner-up” at ACM/IEEE IPSN 2021.
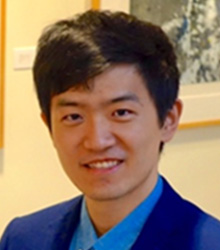
Topic: Millimeter-scale Movement Tracking with Magnetic Sensing

Dongyao Chen
Assistant Professor, Shanghai Jiao Tong University
Abstract:Fine-grained body movements can provide valuable insights into an individual's behavior, including their health status, athletic performance, and human-computer interactions. However, traditional methods for analyzing these movements, such as cameras, RF signal, and inertial measurement units, have limitations when it comes to fine-grained tracking. In this talk, I will introduce two projects of a new technology stack – magnetic sensing – to overcome these limitations and achieve more precise behavioral analysis. The first project focuses on enabling untethered, energy-efficient sensing for behavioral analysis. I will present a systematic approach that allows for accurate monitoring of subtle body movements. Our system can achieve millimeter-level accuracy while only incurring milliwatts of energy overhead. The second project addresses the core challenge of calibrating MEMS magnetometers. By eliminating the need for time-consuming calibration, this system streamlines the process of magnetic sensing and makes it more accessible for a wider range of applications. Through these examples, I will demonstrate the robustness and accuracy of magnetic sensing and highlight its transformative potential for a variety of real-world applications.
BIO:Dongyao Chen is an Assistant Professor of Computer Science at Shanghai Jiao Tong University (SJTU). His research interests are centered around mobile sensing, cyber-physical systems, and security and privacy issues in these areas. He leads the CyPhy team at SJTU, where he and his team work on developing practical systems to solve real-world challenges, particularly in the domains of at-home healthcare and next-gen transportation. His research has been published in top conferences such as MobiCom, MobiSys, UbiComp, CCS, and CoNEXT. Recently, he was awarded the Best Paper Award at VehicleSec 2023. Before joining SJTU as faculty, Dongyao obtained his Ph.D. in Computer Science and Engineering and M.S. in Electrical Engineering from the University of Michigan, Ann Arbor, in 2020 and 2015, respectively. He completed his undergraduate studies at SJTU in 2013.
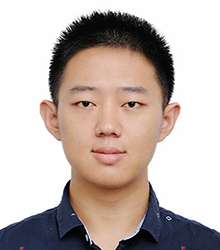
Topic: Carbon emissions and sustainability of 5G mobile networks in China

Tong Li
Assistant Researcher,Tsinghua University
Abstract:5G telecommunication plays a vital role in our daily life and the global economy. However, the energy consumption and carbon emissions of 5G mobile networks are concerning. In this talk, we will introduce a large-scale data-driven framework to quantitatively assess carbon emissions of 5G mobile networks in China, where over 60% of the global 5G base stations are implemented. We reveal a carbon efficiency trap of 5G mobile networks leading to additional carbon emissions of 23.82 megatons in China, caused by the spatio-temporal misalignment between cellular traffic and energy consumption in mobile networks. To address this problem, we propose an energy-saving method, called DeepEnergy, leveraging collaborative deep reinforcement learning and graph neural networks, to make it possible to effectively coordinate the working state of 5G cells, which could help over 71% of Chinese provinces avoid carbon efficiency traps. The application of DeepEnergy can potentially reduce 20.90 megatons of carbon emissions at the national level in 2023. Furthermore, the mobile network in China could accomplish more than 50% of its net-zero goal by integrating DeepEnergy with solar energy systems. Our study deepens the insights into carbon emission mitigation in 5G networks, paving the way towards energy-efficient and sustainable telecommunication infrastructures.
BIO:Dr. Tong Li is a Research Assistant Professor in the Department of Electronic Engineering at Tsinghua University. He received dual PhDs in computer science from the University of Helsinki and in computer science and engineering from the Hong Kong University of Science and Technology in 2021. His primary research interests include wireless network digital twins, network simulation and optimization, and urban computing. Dr. Li has an extensive publication record, with over 40 papers published in renowned journals and conferences such as Nature Sustainability, KDD, WWW, UbiComp, CIKM, Proceedings of the IEEE, TMC, TKDE, and IEEE COMST. Currently, he is responsible for a sub-project of the national key research and development program of China. He has also been awarded the international postdoctoral exchange fellowship program (Talent-Introduction Program), the China postdoctoral science foundation, and the Chinese government award for outstanding self-financed students abroad. Dr. Li is actively involved in the academic community and serves as a program committee member of AAAI, IJCAI, and ECAI.